Take a second to imagine that all of your programming is being audited.
As part of this process, you have to show evidence for how and why you do things the way you do. How much evidence do you have?
You might be able to show data about student learning outcomes or event attendance, but what about the effectiveness of that poster campaign you ran? Or the switch from email communication to text messages?
If you’re like me, you’re always trying to make changes to improve student engagement, but how frequently are we basing what we do in a structured experiment rather than simply on a hunch?
The problem with not testing is that our judgment of our own success can be clouded by our biases.
For example, you might have a number of students come to you and ask for the event request process to be changed. After listening to their reasoning it might seem as though their suggested recommendations will improve engagement, but without testing the impact of these changes you can’t know how it affects the remaining student population.
If you only listen to the feedback from students who come directly to you, it might seem as though the changes have been effective, but there may be a large group of students who have been alienated by the process who might not speak to anyone about it.
This is why it’s so important to measure the impact of our work — not only for the benefit of the students but to make your life easier too! And we already have a framework that we can use to test our hypotheses: The scientific method.
The Scientific Method
This might feel like an episode of “Are You Smarter Than a 5th Grader,” but stick with me on this! Besides, the fact the scientific method is embedded into the elementary curriculum shows just how critical it is to the way we conduct research.
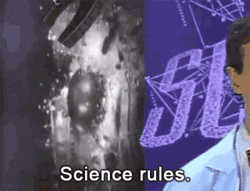
No matter what the movies may show us, using the scientific method doesn’t require lab coats or clipboards. We can use the principles of the scientific method in our day-to-day student affairs work to help analyze and improve the impact of our work.
The scientific method is broken up into 6 steps: purpose, research, hypothesis, experiment, analysis, and conclusion. Let’s use an example to see how this works in practice:
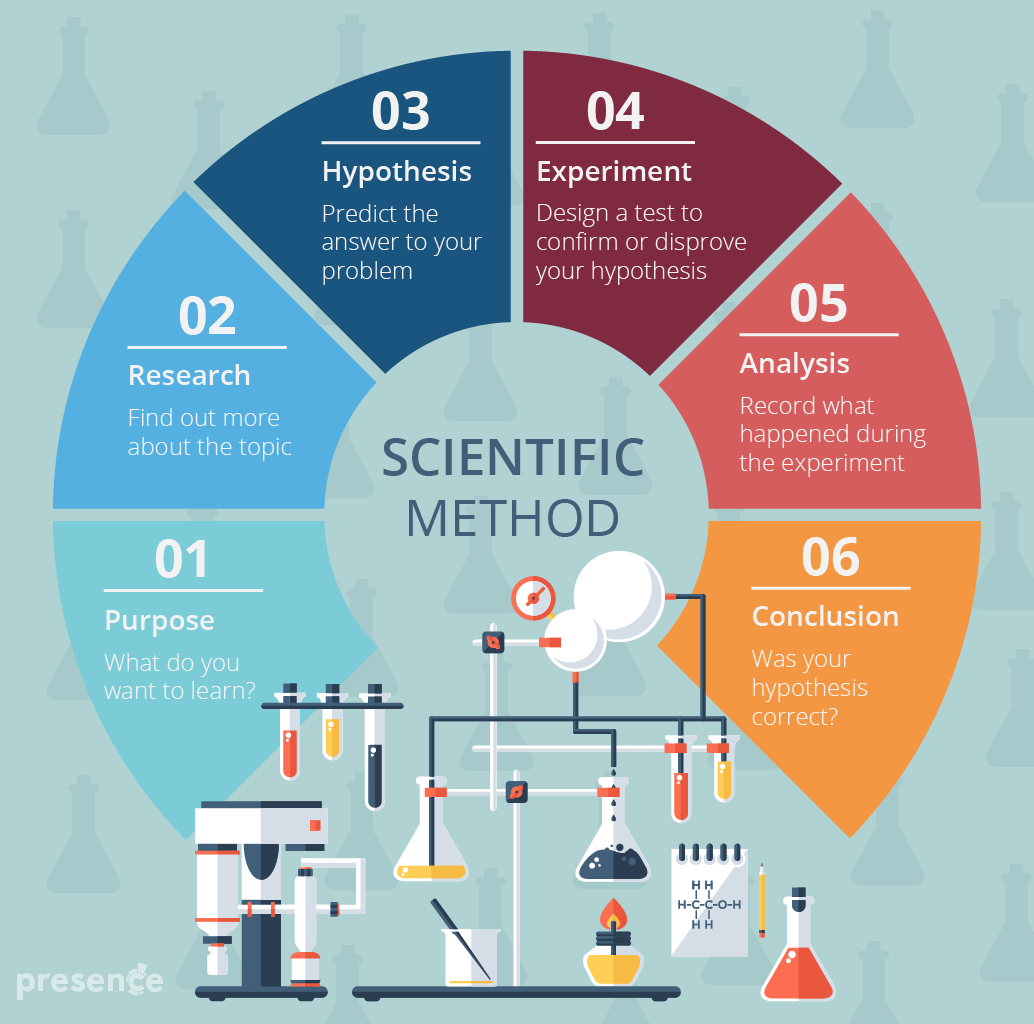
1. Purpose:
This one should be pretty straightforward. Simply, what is it that you want to learn? In this example, let’s say: “To improve the marketing strategy for student events.”
2. Research:
You’ll want to spend time finding resources and/or evidence that support the basis of your experiment. You can use the search function on the student affairs Facebook groups to look through post history or find blogs, Quora posts, or academic articles that are relevant to the subject.
3. Hypothesis:
The hypothesis should be a specific statement that you can measure to prove either true or false. In this instance, that would be something like: “Utilizing digital signage will increase student event attendance.”
4. Experiment:
Here comes the fun part — running your experiments! In this example, you could test using digital signage to promote an event by using it on one campus but not another. You could also test it across two similar events where only one uses the signage. Remember, for any experiment you should always have one control case where the status quo is maintained so that the data can be compared against it.
5. Analysis:
Time to see if your experiment had a significant change. Using event check-in data, you can see if students from one campus or control group had a higher attendance rate. For more accurate data, you might want to repeat this experiment a few times or perform a statistical test to make sure the correlation is related to the variable you’re testing.
6. Conclusion:
Now that you’ve analyzed your data, you’re ready to make a conclusion on your hypothesis. If you found your hypothesis to be true — that advertising with digital signage increased student attendance at your event — now would be the time to present your findings as evidence to make a permanent change.
A/B Testing
For communication like newsletters, web pages, or social posts, using A/B testing (sometimes known as split-testing) is a great way to measure your potential reach. This method uses two test cases to see which performs better with an audience.
Let’s take the example of a newsletter you send to students. There are a lot of variables that factor into an email open rate or click-through rate, like the day of the week, time of day, sender, and subject line.
To A/B test the newsletter, you could create two different subject lines for the same email and send them to a small sample of students. After measuring which one had the better open rate, you can send the better performing email to the remainder of the group.
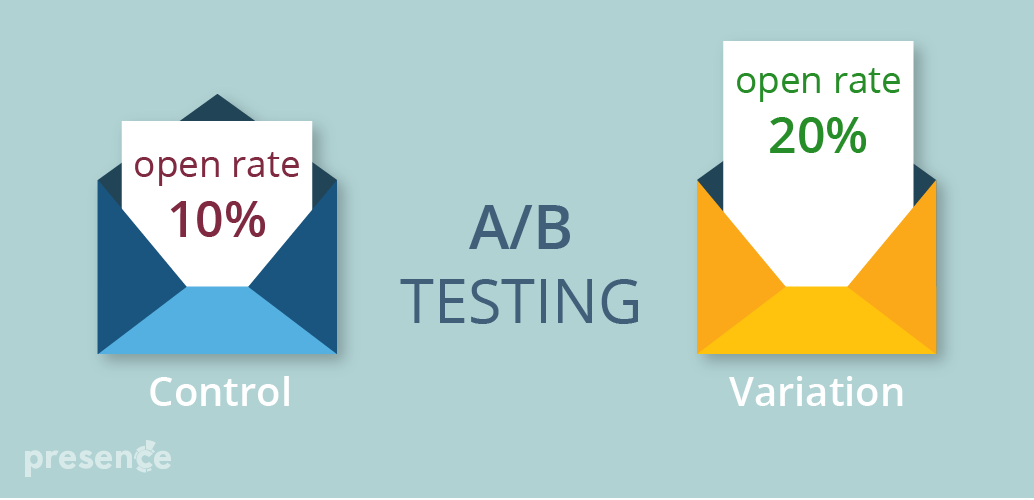
Another way of A/B testing is to segment your audience. For instance, you might break up your email distribution list into students living on campus vs students who commute to campus. You could then send the same email to a sample of each segment once in the morning and once in the evening. This would help determine whether a certain audience type prefers their emails at a certain time of day.
You could modify this test to use in other circumstances such as the design of a poster. For example, if you wanted to see whether the size, imagery, or color use makes a difference on attendance you could post two different versions and poll students after the event to see which one influenced their decision to attend.
Using SIS Data
If you want to take your analysis to the next level, you could integrate all your student touchpoints with data from your student information system (SIS). By doing this, you can see which students are engaging (or not engaging) with your programming.
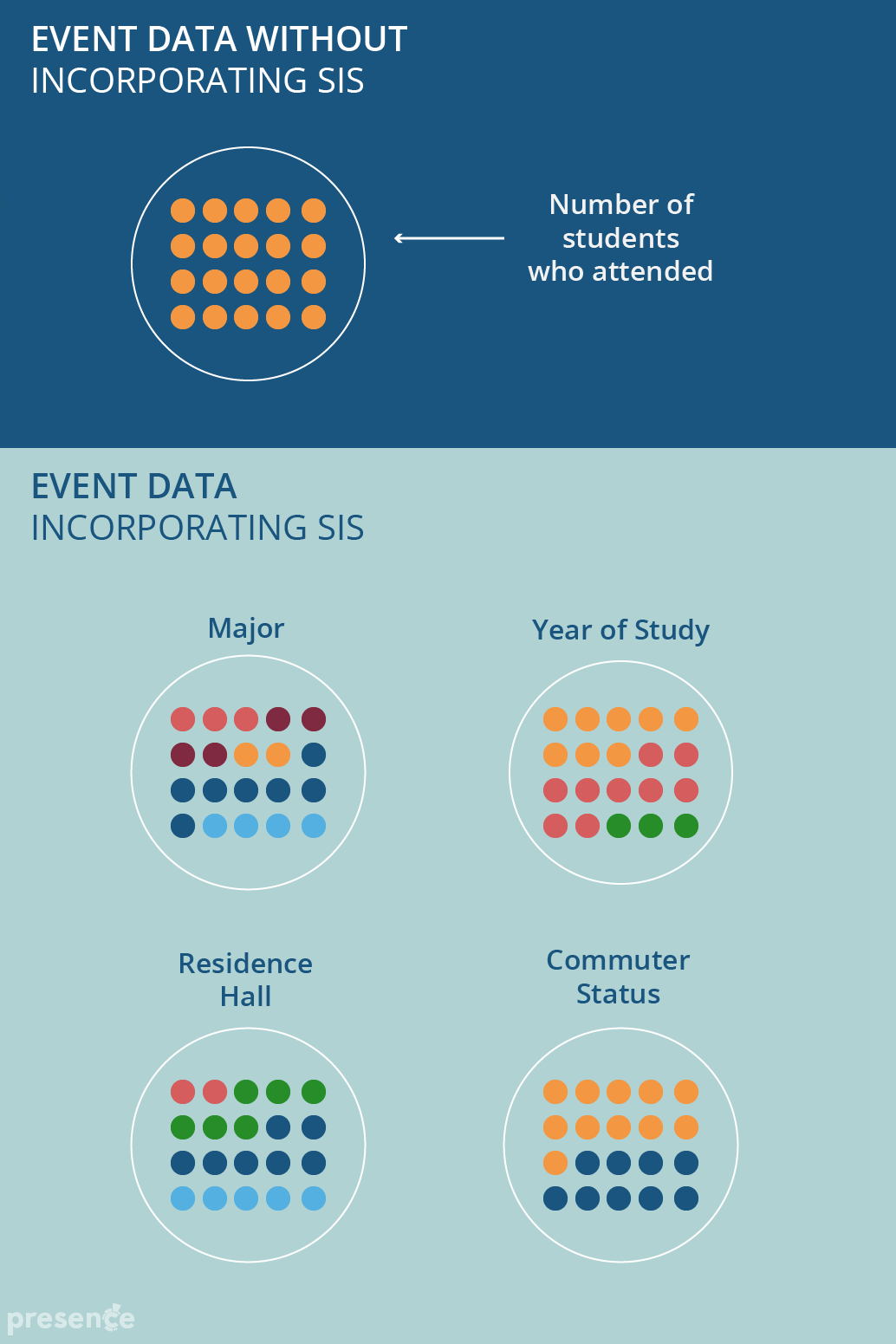
You might be surprised to find a significant difference in engagement from a group of students you didn’t think to measure. First-year STEM students might be less likely to engage in campus events after 5 PM, or students from a particular residence hall may be less likely to sign-up for civic engagement opportunities.
Basic Statistics
For the most part, you’re probably going to be happy to use basic descriptive statistics for your experiments. This is the mean, median, mode, and range. But if you need to really prove your work is having an impact, you might want to start looking at more detailed statistical tests.
For anything where you’re measuring an effect on a single person or group, you’re probably only going to need to use something like a paired t-test. There are lots of helpful resources online on how to do this and you don’t need anything more advanced than a spreadsheet like Excel or Google Sheets. These type of tests are particularly popular with schools who are assessing the impact of training on students.
The Benefits
Investing time in experimenting and analyzing data can seem like a big commitment, but actually, there are a ton of short-term and long-term benefits that will make your experimentation totally worth it.
Funding
Data gathered from assessment helps to justify spending by providing evidence of return on investment (ROI) for various departmental and divisional budgets. This data can also be used to argue for more resources by spotting areas that need more funding and that aren’t performing up to par.
Efficiency
Experimentation and analysis can identify areas that present a relatively low impact compared to the amount of effort you invest in them. Reallocating time and resources to higher impact areas creates a more efficient department and a less stressed you. Collecting data on efficacy can also benefit departments other than your own. By sharing assessment data, departments can collaborate on projects and spend less time duplicating efforts to uncover extant information.
Engagement
Assessing the people who attend programs is important, but complete data sets will also allow you to see who is not engaged, and therefore will allow you to re-strategize and develop programs and marketing systems that reach a larger group of your targeted students. This is one of the ways that you can develop intervention strategies and improve retention.
Assessment
We’ve written extensively about creating learning outcomes, and assessing them is important, too. There are tons of ways to assess how students are fulfilling learning outcomes, the efficacy of your programs, and what areas on campus need to be further developed. For some helpful resources, check out this blog post by Joe Levy and this comprehensive e-book we wrote.
Retention
Retention is improved by creating intervention strategies that spot when students are likely to drop out. Lynn University combined student involvement data with proactive intervention strategies, and the results they found validate all of the work you’re doing on campus.
The real takeaway here is that we should always be looking to improve our work in a systematic, measurable way. Measuring changes by anecdotal feedback or unstructured observation may only serve to reinforce our own biases. Having data to back-up our improvements will serve as a gateway to future funding, efficiencies, and engagement.
Are you ready to break out the scientific method on your campus? Make sure to let us know how it goes by tweeting us @themoderncampus!